Generative AI - A Revolution in Hardware Design and Robotics
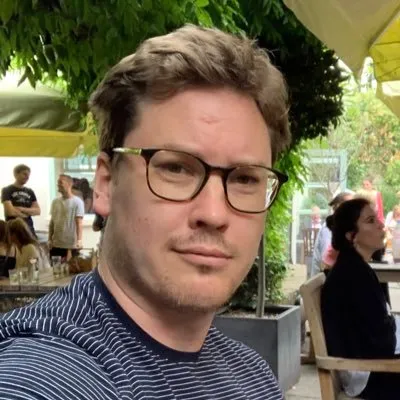
The Evolution of Generative AI
Generative AI has undergone significant transformation over the last decade. From its rudimentary capabilities of crafting simplistic statistical patterns, it has evolved into a robust tool capable of generating compelling music, intricate artwork, and convincing text.
A central player in this evolution has been the development of neural networks and deep learning. This new approach, inspired by the structure of the human brain, allowed generative AI to create more complex and realistic outputs. The introduction of Generative Adversarial Networks (GANs) in 2014 by Ian Goodfellow marked a significant turning point in the sophistication of generative AI models. Comprising a generator network producing data and a discriminator network evaluating the quality of this data, GANs engage in a continuous learning cycle, each striving to outdo the other. This revolutionary framework enabled the creation of incredibly realistic images and paved the way for transformative change.
Variational Autoencoders (VAEs), another crucial model type, further contributed to the evolution of generative AI. Unlike GANs, which can sometimes produce irregular results due to their adversarial setup, VAEs ensure a more stable and consistent output, adding another dimension of control and reliability to the generative process.
In recent years, transformer-based models, such as OpenAI's GPT (Generative Pre-trained Transformer) series, have caused ripples across the AI landscape. Trained on vast quantities of text data, these models demonstrate an uncanny ability to generate text that's indistinguishable from human writing. This capability has practical applications ranging from content generation to customer service automation and beyond.
Despite these significant advances, the evolution of generative AI is far from complete. Generative models, though capable of producing stunningly realistic results, still struggle with context understanding and maintaining long-term coherence in their outputs. Moreover, the ethical implications and potential misuse of this technology require careful scrutiny and regulation. Looking to the future, the development of generative AI will likely continue to be shaped by advancements in machine learning, increasing computational power, and the availability of large, high-quality datasets.
Challenges in Hardware Design and Robotics
While the application of generative AI in hardware design and robotics is still in its early stages, the potential benefits are substantial. The complex nature of these fields, however, presents several challenges.
In hardware design, the diversity of the components and their varying interactions complicate AI’s understanding. Each component, from microprocessors to power units, has unique physical properties and operational constraints. An AI model would need to consider all these variables to successfully design a functional piece of hardware. This is a far cry from simpler applications such as text or image generation, where data is more uniform, and the generative process is less influenced by external constraints.
In robotics, the challenge intensifies. Robotic systems interact with the physical world and are therefore subject to a vast array of environmental variables. These can range from physical laws like gravity and friction to unpredictable factors like weather conditions and human interaction. Training an AI to navigate this labyrinth of potential scenarios requires substantial data and advanced learning models, both of which are still maturing in the field of robotics.
Moreover, data scarcity poses another challenge. While there's a wealth of text, music, and images readily available for training AI, datasets in hardware design and robotics are less abundant. They often exist in proprietary silos within tech companies or research labs. And even when available, these datasets are complex and multi-dimensional, requiring a deep understanding of the underlying principles of physics, material science, and engineering.
Lastly, the safety and reliability standards in hardware design and robotics are exceptionally high. The stakes of an error can be significant, from the malfunction of critical infrastructure to the failure of a robotic surgical tool. This necessity for near-perfect performance demands advanced testing and validation methodologies that generative AI is still catching up to.
The Symbiosis of AI and Humans in Complex Fields
Despite these challenges, there's significant potential in combining the strengths of AI and humans to push the boundaries of what's possible in complex fields like hardware design and robotics.
While AI offers the capacity to process vast amounts of data and identify patterns, humans bring the creativity and experience required for complex problem-solving and decision-making. It's this blend of brute computational force and nuanced human insight that can truly propel progress.
In hardware design, for instance, generative AI could automate and optimize component layout or circuit routing tasks, which are traditionally time-consuming and tedious. This would allow engineers to focus their efforts on higher-level design problems, such as architecting more energy-efficient or powerful devices.
In robotics, AI could assist with design optimization and control strategy formulation. By simulating and testing different designs and strategies, AI could save countless hours of physical prototyping and testing. Human engineers, meanwhile, could focus on refining these designs or strategies, applying their intuition, and troubleshooting unforeseen issues.
This AI-human collaboration also extends to the validation and safety aspects. While AI algorithms can conduct preliminary tests and identify potential design flaws or failure points, human experts are crucial for interpreting these results, understanding their real-world implications, and making final decisions.
Moreover, humans play a vital role in setting the objectives and ethical boundaries for AI. This is particularly important in fields like hardware design and robotics, which have profound societal and environmental impacts. Guiding AI towards sustainable and responsible design choices is a responsibility that falls squarely on human shoulders.
The successful integration of AI in these fields will not only enhance productivity but also open up new avenues for innovation. As the technology matures and these collaborative models become more prevalent, we can expect to see increasingly sophisticated hardware designs and robotic systems – a testament to the power of AI-human symbiosis.
The Shift in Human Resource Allocation
As the role of AI in routine tasks continues to expand, we are standing at the precipice of a paradigm shift in human resources. It is clear that we are moving towards a future where human skills will be required for more complex, creative, and interpretive tasks, while AI and automated systems handle the mundane and repetitive.
This shift has a two-fold impact: on the one hand, job roles that largely involve routine tasks risk becoming obsolete. On the other hand, a range of new roles are likely to emerge, ones that involve guiding AI systems, interpreting their outputs, and even 'training' these systems to perform their roles better.
A prime example of such a new role would be that of an AI trainer. As AI systems become more sophisticated, they require nuanced, human-like inputs during their training phase. AI trainers are professionals who work with AI systems during their 'learning' phase, providing feedback and improving their performance.
In a similar vein, the demand for AI ethics specialists is likely to surge. As AI systems increasingly interact with humans and make decisions that affect human lives, the need for these systems to make ethically sound decisions becomes paramount. AI ethics specialists would be responsible for guiding the ethical frameworks within which these AI systems operate.
This shift will also impact the required skill sets for many professions. Skills that AI systems find hard to replicate — such as critical thinking, creativity, emotional intelligence, and leadership — are likely to be in higher demand. Additionally, AI literacy — a general understanding of how AI systems work and their societal implications — will become a necessary skill in an increasingly AI-driven world.
Educational Adaptations for an AI-Augmented Future
This shift in human resource allocation will necessitate a corresponding shift in education. Preparing the next generation for an AI-augmented future will require integrating AI literacy into education at all levels.
From primary education through to higher education, a greater emphasis will need to be placed on computational thinking, understanding the basics of AI, and ethical considerations around AI use. Students will need to understand not just how to use AI as a tool, but how to work alongside AI, how to harness its strengths, compensate for its weaknesses, and navigate the ethical minefields it presents.
Moreover, as AI begins to take over more routine tasks, problem-solving skills will be more crucial than ever. The ability to handle ambiguity, interpret complex situations, and come up with innovative solutions will be highly sought-after skills in the job market. Therefore, pedagogical strategies that encourage active learning, creativity, critical thinking, and problem-solving will be instrumental in shaping a future-ready workforce.
This transformation in education will not be without its challenges. Questions around access to technology, teacher training, curriculum development, and student engagement will need to be carefully navigated. But the rewards — a generation of students prepared for an AI-augmented future — are worth striving for.
Future of Generative AI
The horizon of generative AI, particularly in intricate fields like hardware design and robotics, promises a future that is as exciting as it is daunting. Progress in AI methodologies, along with a growing wealth of data, point to a promising trend of improvements in AI's capacity to learn from and generate complex hardware designs or robotic systems. But, as we move towards this future, we must acknowledge that the journey is not solely about technological advances; it also demands comprehensive understanding, effective regulation, and meaningful human engagement.
In the future, generative AI could transform the way we approach hardware design and robotics. Imagine a world where AI systems design and test new microprocessors, where they recommend optimizations for energy efficiency or create novel robotics control strategies. The complex task of hardware design could be dramatically expedited, and the possibilities for innovation in robotics could multiply.
However, while these advances paint an optimistic picture, it is crucial to take a balanced view. A technology-centric perspective is insufficient to grapple with the full range of implications that these developments could bring about. An understanding of the wider socio-economic, ethical, and legal ramifications will be essential. These considerations should inform the regulations that will be necessary to ensure the safe, ethical, and effective use of generative AI. These regulations will need to be dynamic, evolving alongside the technology and societal needs.
Moreover, the future of generative AI should not be one where AI replaces humans but one where it works alongside them. The unique strengths of human creativity, intuition, and ethical judgment must complement AI's ability to handle vast data and complex computations. This symbiosis is paramount in realizing the potential of generative AI. The path forward will require thoughtful human oversight and intervention, which will not only guide the development of the technology but also ensure that its deployment aligns with our values and societal goals.
In essence, the future of generative AI is vast in potential. But realizing it will require more than just technological innovation. It calls for a vision that recognizes the value of human-AI collaboration, that understands the broader implications of the technology, and that appreciates the importance of thoughtful oversight and regulation.
As we advance into this future, we must remember that technology is merely a tool. It is how we use it, how we guide it, and how we adapt to it that will shape our world. As we stand on the brink of a new era in generative AI, it is up to us to ensure that it is a future that brings prosperity, justice, and innovation, while preserving the uniqueness of the human experience.
The promises of generative AI are vast, reshaping our world in ways we are only beginning to comprehend. With the right blend of human ingenuity and AI's capabilities, the horizon of generative AI promises a future that is as fascinating as it is promising.