Ollama on Raspberry Pi 5
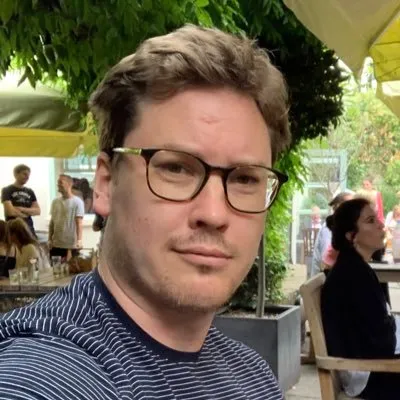
Ben
@benjislab
This guide demonstrates how to deploy generative AI models locally on a Raspberry Pi using Ollama, an open-source framework for edge AI. You'll learn to:
- Install Ollama on Raspberry Pi OS (64-bit)
- Run optimized models like TinyLlama (1.1B parameters) and Llama3 (8B)
- Integrate models via REST API with error handling
- Benchmark performance across hardware configurations
- Build real-world applications from chatbots to content generators
Key advantage: Process sensitive data locally without cloud dependencies.
Prerequisites
Hardware
- Raspberry Pi 5 (8GB RAM recommended)
- 32GB+ U3 SD Card (A2-class for better I/O)
- Active cooling solution (heatsink + fan)
- Official Raspberry Pi 5 power supply (5V 5A) or equivalent USB-C PD power supply
Software
- Raspberry Pi OS 64-bit (Bookworm)
- Ollama v0.1.20+
# First-time setup
sudo apt update && sudo apt full-upgrade -y
sudo apt install curl git python3-venv
Why Ollama on Raspberry Pi?
- Privacy: No data leaves your device
- Cost: Avoid cloud API fees
- Latency: <1s responses with quantized models
- Education: Experiment with LLMs hands-on
Example Use Case: A hospital uses Ollama-powered Pis to anonymize patient notes locally, reducing HIPAA compliance risks.
Installation Guide
1. Install Ollama
# Download and verify install script
curl -fsSL https://ollama.com/install.sh | sh
# Enable service (auto-start on boot)
sudo systemctl enable ollama
# Verify installation
ollama --version # Should return v0.1.20+
2. Select Your Model
Model | Size | RAM Use | Best For |
---|---|---|---|
TinyLlama | 1.1B | 400MB | Quick Q&A, simple tasks |
Llama3-8B | 8B | 4.8GB | Complex reasoning |
Phi-2 | 2.7B | 1.5GB | Coding assistance |
# Download TinyLlama (1.1B parameters)
ollama run tinyllama
# For advanced tasks (requires 8GB Pi):
ollama pull llama3:8b
API Integration
Basic Query
curl http://localhost:11434/api/generate -d '{
"model": "tinyllama",
"prompt": "Explain quantum computing in 50 words",
"stream": false,
"options": { "temperature": 0.7 }
}'
Sample Response:
{
"response": "Quantum computing uses qubits...",
"created_at": "2025-03-24T09:00:00Z",
"total_duration": 420
}
Python Client
import requests
def query_ollama(prompt: str, model: str = "tinyllama") -> str:
try:
response = requests.post(
"http://localhost:11434/api/generate",
json={"model": model, "prompt": prompt, "stream": False},
timeout=10
)
return response.json()["response"]
except requests.exceptions.RequestException as e:
return f"API Error: {str(e)}"
Performance Optimization
Benchmark Results (Pi 5 8GB)
Metric | TinyLlama | Llama3-8B |
---|---|---|
Load Time | 2.1s | 18.4s |
Tokens/Second | 42.7 | 9.2 |
RAM Usage | 412MB | 4.9GB |
Power Draw | 3.8W | 7.1W |
Optimization Tips:
1. Add swap space:
sudo dphys-swapfile swapoff
sudo nano /etc/dphys-swapfile # Set CONF_SWAPSIZE=2048
sudo dphys-swapfile setup && sudo dphys-swapfile swapon
2. Use model quantization:
ollama run llama3:8b-q4_0 # 4-bit quantized version
Real-World Applications
1. Offline Chatbot
while True:
user_input = input("You: ")
if user_input.lower() == "exit":
break
print("AI:", query_ollama(user_input))
2. Document Analyzer
ollama run llava:13b # Multi-modal model for image+text
3. API Server
# Expose Ollama to local network (caution!)
ollama serve --host 0.0.0.0
Troubleshooting
Issue | Solution |
---|---|
OutOfMemory errors | Use smaller model, add swap |
Slow responses | Quantize model, disable background tasks |
Installation failures | Check ARM64 compatibility, reflash OS |
Conclusion
Ollama transforms Raspberry Pi into a portable AI workstation. While current models can't match cloud-scale GPT-4, they enable:
- Private data processing
- Educational prototyping
- Low-cost edge deployments
As open-source models improve (Mistral, LLaMA-3), expect Pi-powered AI kiosks, IoT controllers, and offline assistants to proliferate.